Unveiling the Impact of Generative AI on Accelerating Innovation in Drug Discovery
Generative AI is revolutionizing the field of drug discovery, offering unprecedented opportunities to accelerate the development of new treatments. Traditionally, discovering and developing new drugs is a lengthy and costly process, often taking years of research and billions of dollars in investment. However, the integration of generative AI models has transformed this landscape by enabling researchers to simulate complex molecular structures, predict potential drug candidates, and optimize chemical compositions with greater speed and accuracy.
Fig: Generative AIAI-powered algorithms can sift through vast datasets, identify patterns, and suggest novel compounds that would be difficult for humans to uncover alone. This technology not only reduces the time needed for preclinical research but also improves the precision of drug design, increasing the likelihood of successful outcomes in clinical trials. Furthermore, AI’s ability to adapt and learn continuously means that it can evolve alongside emerging biological challenges, such as new diseases or resistant strains. As a result, generative AI is poised to be a game changer in the pharmaceutical industry, driving faster, more efficient drug discovery processes.
Table of Content
What is Generative AI?
The Intersection of AI and Drug Discovery
Key Benefits of Using Generative AI in Drug Discovery
Role of Generative AI in Drug Discovery Process: A Step-by-Step Procedure
AI-Driven Drug Repurposing
Challenges in the Traditional Drug Discovery Process
Future Trends in AI-Driven Drug Discovery
ConclusionWhat is Generative AI?
Generative AI is a branch of artificial intelligence that focuses on creating new data, content, or solutions by learning patterns from existing data. Unlike traditional AI, which classifies or analyzes information, generative AI models are capable of producing original outputs such as text, images, music, or even complex molecular structures. These models, often based on neural networks like Generative Adversarial Networks (GANs) or transformers, are trained on large datasets and then use that knowledge to generate realistic or novel results.
For example, in drug discovery, generative AI development can simulate the design of new compounds by predicting chemical structures that have the potential to interact with biological targets, speeding up the research and development process. Its applications are vast, ranging from creative industries to scientific fields, and it is rapidly transforming how problems are approached in sectors like healthcare, gaming, and finance. By pushing the boundaries of machine learning, generative AI opens up new possibilities for innovation and problem-solving in a wide variety of domains.
The Intersection of AI and Drug Discovery
The intersection of AI and drug discovery is reshaping the pharmaceutical industry, offering cutting-edge tools to tackle the complexities of developing new treatments. AI, particularly machine learning and generative models enables researchers to analyze massive datasets, identify potential drug candidates, and predict how compounds will interact with biological targets. This technology significantly accelerates early-stage research, where traditional methods can take years and cost millions.
By using AI to simulate molecular interactions, optimize chemical structures, and streamline the identification of lead compounds, drug discovery becomes faster, more cost-effective, and more precise. AI also helps in personalizing treatments by analyzing genetic, clinical, and molecular data to design drugs tailored to individual patient needs.
Additionally, it aids in repurposing existing drugs for new therapeutic areas, thus maximizing efficiency. As AI continues to evolve, its integration with drug discovery is expected to deepen, unlocking new possibilities for medical innovation and revolutionizing the way we approach healthcare and treatment development in the future.
Key Benefits of Using Generative AI in Drug Discovery
Generative AI offers numerous benefits in drug discovery, transforming traditional approaches and significantly enhancing the efficiency and effectiveness of the process. Here are some key benefits:
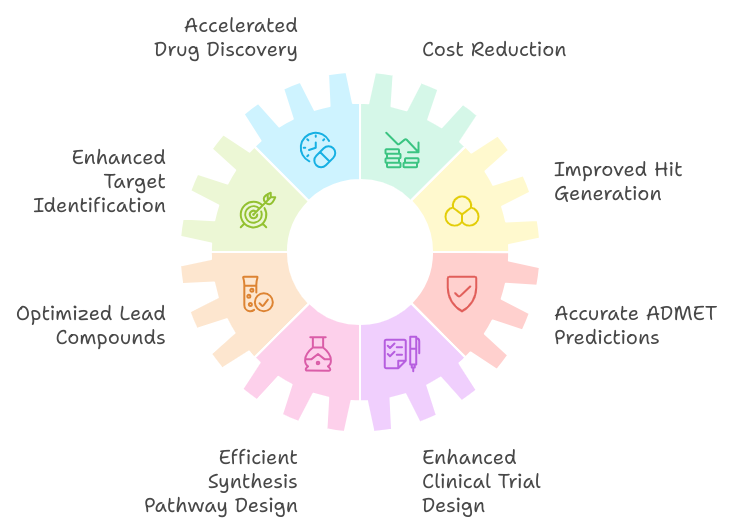
1. Accelerated Drug Discovery
- Benefit: Generative AI speeds up the drug discovery process by rapidly generating and evaluating new chemical compounds.
- Impact: This acceleration reduces the time needed to identify promising drug candidates, allowing for faster progression from concept to clinical trials.
2. Cost Reduction
- Benefit: AI reduces the costs associated with drug discovery by minimizing the need for extensive laboratory testing and experimental work.
- Impact: Lower costs make drug development more accessible and economically viable, particularly for smaller biotech firms and startups.
3. Enhanced Target Identification
- Benefit: AI can analyze large-scale biological data to identify and validate new drug targets more efficiently than traditional methods.
- Impact: Improved target identification increases the likelihood of discovering novel therapeutic avenues and effective treatments.
4. Improved Hit Generation
- Benefit: Generative AI creates novel chemical structures and predicts their interactions with drug targets, leading to the identification of new hits.
- Impact: The ability to explore a vast chemical space accelerates the discovery of potential drug candidates with high efficacy.
5. Optimized Lead Compounds
- Benefit: AI models optimize lead compounds by predicting how structural modifications will impact their properties, such as potency, selectivity, and safety.
- Impact: Enhanced lead optimization improves the chances of developing effective and safe drugs, reducing the likelihood of late-stage failures.
6. Accurate ADMET Predictions
- Benefit: Generative AI predicts Absorption, Distribution, Metabolism, Excretion, and Toxicity (ADMET) properties early in the drug discovery process.
- Impact: Early prediction of ADMET properties helps filter out compounds with undesirable profiles, saving time and resources in preclinical testing.
7. Efficient Synthesis Pathway Design
- Benefit: AI assists in designing efficient and scalable synthesis pathways for drug candidates, streamlining the chemical manufacturing process.
- Impact: Improved synthesis design accelerates the production of drug candidates and reduces costs associated with chemical synthesis.
8. Enhanced Clinical Trial Design
- Benefit: AI optimizes clinical trial design by predicting optimal dosages, identifying suitable patient populations, and simulating trial outcomes.
- Impact: More effective trial design increases the likelihood of clinical success and reduces the resources needed for trial execution.
9. Drug Repurposing Opportunities
- Benefit: AI identifies new uses for existing drugs by analyzing patterns in drug interactions and disease data.
- Impact: Faster and cost-effective identification of new therapeutic indications for existing drugs, potentially addressing unmet medical needs.
10. Personalized Medicine
- Benefit: AI enables the development of personalized treatments by analyzing patient-specific data and tailoring drug candidates to individual profiles.
- Impact: Personalized medicine improves treatment efficacy and reduces adverse effects, leading to better patient outcomes.
11. Real-World Data Integration
- Benefit: AI leverages real-world data (RWD) from electronic health records and other sources to inform drug discovery and development.
- Impact: Integration of RWD provides insights into drug efficacy and safety in diverse patient populations, enhancing the relevance of clinical trials.
12. Continuous Learning and Improvement
- Benefit: Generative AI systems continuously learn from new data and outcomes, improving their predictions and recommendations over time.
- Impact: Ongoing learning enhances the accuracy and reliability of AI-driven drug discovery, leading to better results and more innovative solutions.
13. Increased Innovation
- Benefit: AI enables the exploration of novel chemical spaces and therapeutic targets that may not be accessible through traditional methods.
- Impact: This leads to the discovery of innovative drug candidates and potential breakthroughs in treatment.
Generative AI brings transformative benefits to drug discovery by accelerating the process, reducing costs, and enhancing various stages of drug development. Its ability to analyze vast amounts of data, generate novel compounds, and optimize drug properties makes it a powerful tool for advancing pharmaceutical research and improving patient outcomes.
Role of Generative AI in Drug Discovery Process: A Step-by-Step Procedure
Generative AI is transforming the traditional drug discovery process by making it faster, more cost-effective, and more efficient. By leveraging AI’s ability to analyze vast datasets, generate novel chemical compounds, and predict drug interactions, generative AI can significantly accelerate each phase of the drug discovery pipeline.
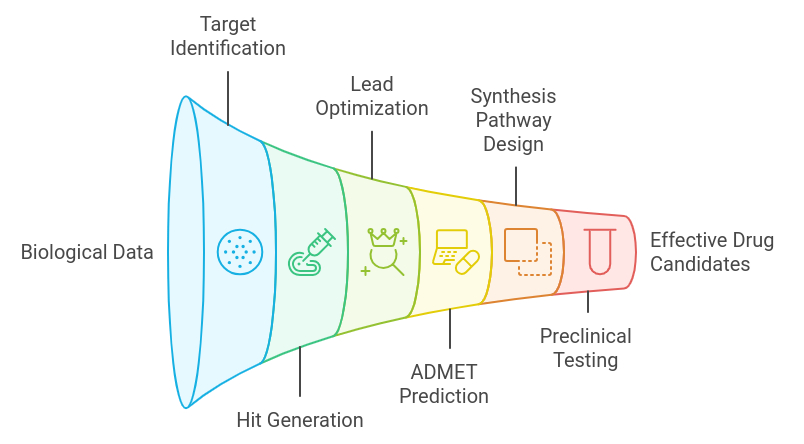
Here’s a step-by-step look at how generative AI plays a role in the drug discovery process:
1. Target Identification
- Traditional Approach: Identifying the biological targets (such as proteins or genes) that are linked to a disease is time-consuming and requires extensive experimentation.
- Generative AI Role: AI models analyze large-scale biological data, including genomic, proteomic, and transcriptomic data, to predict and prioritize potential drug targets. Machine learning algorithms identify novel targets by recognizing patterns that human researchers may miss.
2. Hit Generation (Compound Design)
- Traditional Approach: Researchers rely on libraries of existing compounds and trial-and-error methods to find molecules that interact with the target.
- Generative AI Role: Generative AI models, such as deep learning-based approaches, can generate novel chemical structures that bind to the target more effectively. These AI models simulate possible molecular designs, providing new drug-like compounds that may not exist in current databases. Techniques like Generative Adversarial Networks (GANs) and Variational Autoencoders (VAEs) are used to explore chemical space and propose optimized molecules.
3. Lead Optimization
- Traditional Approach: Optimizing a lead compound for drug-like properties, such as potency, stability, and safety, is often labor-intensive and iterative.
- Generative AI Role: AI models predict how changes in molecular structure impact various properties, such as binding affinity, toxicity, and bioavailability. AI optimizes the compound for multiple parameters simultaneously by running simulations, reducing the need for repeated lab testing.
4. Prediction of ADMET Properties (Absorption, Distribution, Metabolism, Excretion, and Toxicity)
- Traditional Approach: Experimental methods such as animal testing and in vitro assays are typically used to assess ADMET properties, which is costly and time-consuming.
- Generative AI Role: AI models predict ADMET properties early in the drug discovery process by analyzing the molecular structure and running simulations. This helps to filter out compounds that are likely to have undesirable properties, thus saving time and reducing the need for animal testing.
5. Synthesis Pathway Design
- Traditional Approach: Developing a chemical synthesis pathway to create the lead compound is an elaborate process involving manual trial and error.
- Generative AI Role: AI models, trained on existing chemical reaction data, can generate synthesis pathways for new compounds. AI tools like retrosynthesis analysis propose novel, efficient, and scalable ways to synthesize a drug, streamlining the chemical manufacturing process.
6. Preclinical Testing
- Traditional Approach: Drugs undergo extensive testing in animal models to evaluate safety, efficacy, and dosage before entering human clinical trials.
- Generative AI Role: AI can predict the outcomes of preclinical tests by using models trained on historical data. Generative AI reduces reliance on physical preclinical testing by simulating the behavior of the drug in various biological contexts, such as tissue or organ interactions, thus enhancing the accuracy of preclinical decisions.
7. Clinical Trial Design and Optimization
- Traditional Approach: Clinical trials are resource-intensive and often involve significant trial-and-error to determine dosage, patient recruitment, and trial design.
- Generative AI Role: AI-driven models assist in designing clinical trials by predicting optimal dosages, patient populations, and endpoints. It uses historical trial data and patient records to identify the best candidates for trials, improving trial success rates and reducing costs. AI also helps simulate potential outcomes, enabling more targeted trials.
8. Data Analysis and Post-Marketing Surveillance
- Traditional Approach: After a drug is approved, post-marketing surveillance is required to monitor adverse events and gather real-world data, which involves manual data collection and analysis.
- Generative AI Role: AI can quickly analyze real-world data from electronic health records, social media, and other sources to detect potential adverse effects and identify new therapeutic uses for approved drugs. AI continuously monitors drug performance, offering insights for improvements.
Generative AI offers a transformative approach to the drug discovery process by addressing bottlenecks at each stage. Its ability to simulate biological interactions, predict outcomes, and generate novel compounds allows for faster, cheaper, and more effective drug discovery, potentially revolutionizing the pharmaceutical industry.
AI-Driven Drug Repurposing
AI-driven drug repurposing is a groundbreaking approach that leverages generative AI to identify new therapeutic uses for existing medications. By applying advanced generative models, such as deep learning algorithms and neural networks, researchers can analyze extensive biomedical data, including genetic, molecular, and clinical information. These AI systems can uncover hidden patterns and relationships between drugs and diseases that may not be apparent through traditional methods. For instance, generative AI can simulate how a drug designed for one condition might interact with different biological targets, revealing potential new applications.
This approach not only accelerates the drug discovery process but also reduces costs and risks associated with developing new treatments from scratch. By repurposing existing drugs, which have already undergone significant testing for safety and efficacy, researchers can expedite the development of new therapies for unmet medical needs. AI-driven drug repurposing represents a powerful fusion of technology and pharmaceutical research, offering innovative solutions and expanding treatment options in the quest for improved healthcare.
Challenges in the Traditional Drug Discovery Process
The traditional drug discovery process faces several challenges that can impede the development of effective treatments. Some of the key challenges include:
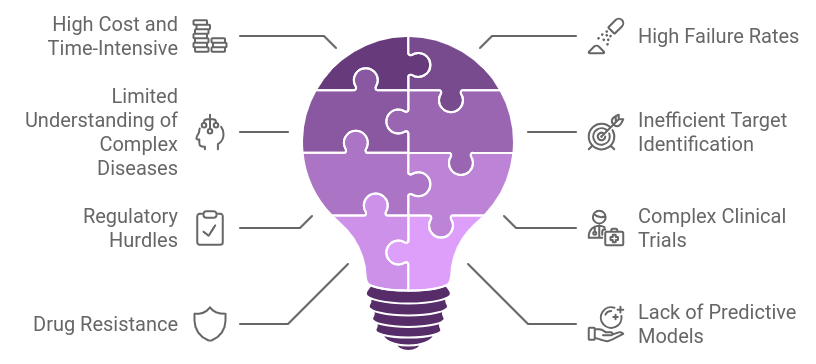
1. High Cost and Time-Intensive
- Cost: Traditional drug discovery is extremely expensive, often requiring billions of dollars and several years to bring a drug to market.
- Time: It can take 10–15 years for a new drug to move through the discovery, development, and approval pipeline, delaying treatment availability.
2. High Failure Rates
- Many drug candidates fail in clinical trials due to poor efficacy or unexpected safety issues. This leads to a significant loss of resources, both financially and in terms of time.
- Only a small percentage of drugs that begin clinical trials receive regulatory approval.
3. Limited Understanding of Complex Diseases
- For diseases like cancer, Alzheimer’s, and autoimmune disorders, the biological mechanisms are highly complex and not fully understood, making drug targeting more challenging.
- This results in a trial-and-error approach, which can delay progress and lead to failure in finding effective treatments.
4. Inefficient Target Identification
- Identifying viable drug targets (biomolecules that drugs can bind to) is often difficult and time-consuming. Errors in early-stage target identification can lead to failure in later stages of drug development.
5. Regulatory Hurdles
- The approval process is long and complex, with stringent regulatory requirements that can delay development.
- Drugs must meet rigorous standards for safety and efficacy, which can be difficult to demonstrate in clinical trials, especially for novel therapeutic classes.
6. Complex Clinical Trials
- Clinical trials, the most resource-intensive part of drug discovery, can be delayed by challenges in patient recruitment, trial design, and adherence to regulatory protocols.
- The need for large-scale trials to demonstrate efficacy across diverse patient populations further complicates the process.
7. Drug Resistance
- In areas such as antibiotics or cancer treatments, resistance to drugs can develop over time, rendering them ineffective and necessitating further discovery efforts.
- This requires constant development of new drugs, which exacerbates the cost and complexity of the process.
8. Lack of Predictive Models
- Preclinical models (like animal testing) often fail to accurately predict how a drug will behave in humans, leading to high attrition rates in clinical trials.
- There is a pressing need for better predictive models to improve drug efficacy and safety outcomes.
9. Intellectual Property and Market Exclusivity
- Securing patents and protecting intellectual property can be difficult, particularly with the growing complexity of biological drugs and biosimilars.
- The competitive market also pressures companies to rush development, sometimes leading to incomplete research or failures.
10. Ethical Considerations
- Human testing in clinical trials poses ethical challenges, especially in areas where experimental treatments are tested on vulnerable populations.
- Balancing patient safety with the need for innovation can slow down the process.
Addressing these challenges often requires new technologies like AI, machine learning, and better computational tools to accelerate and improve the drug discovery process.
Future Trends in AI-Driven Drug Discovery
The future of AI-driven drug discovery is poised to bring significant advancements, driven by ongoing innovations in machine learning, data science, and computational biology. Here are some key trends that are likely to shape the field in the coming years:
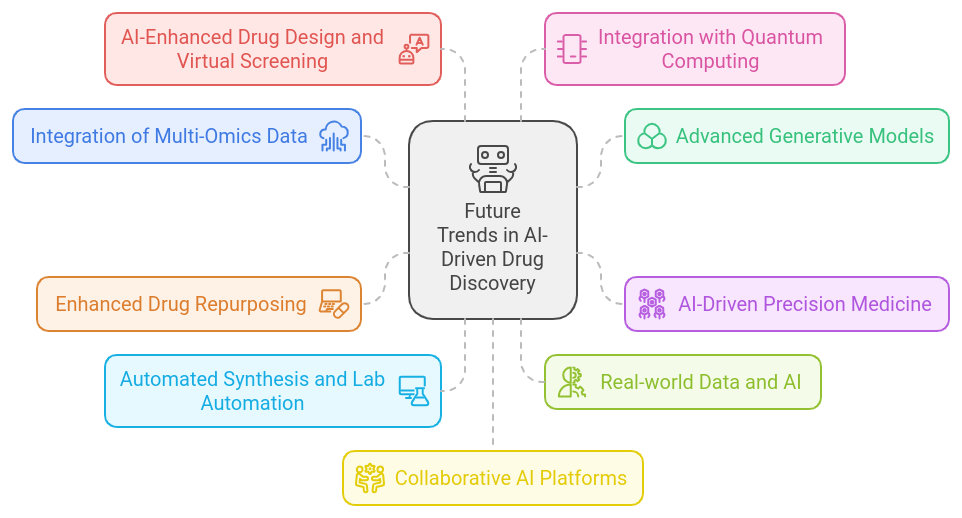
1. Integration of Multi-Omics Data
- Trend: The integration of genomics, proteomics, metabolomics, and other omics data with AI to provide a more comprehensive understanding of disease mechanisms and drug interactions.
- Impact: Enhanced ability to identify novel drug targets, understand complex biological processes, and develop personalized treatments based on a holistic view of the patient’s biology.
2. Advanced Generative Models
- Trend: The development of more sophisticated generative models, such as improved versions of Generative Adversarial Networks (GANs) and Variational Autoencoders (VAEs), to create novel compounds and predict their interactions.
- Impact: Accelerated hit generation and lead optimization, leading to the discovery of innovative drug candidates with higher success rates.
3. AI-Driven Precision Medicine
- Trend: Leveraging AI to tailor treatments to individual patients based on their genetic, environmental, and lifestyle factors.
- Impact: Development of highly personalized drugs that are more effective and have fewer side effects, improving patient outcomes and drug efficacy.
4. Enhanced Drug Repurposing
- Trend: Using AI to identify new uses for existing drugs, can be more cost-effective than developing new drugs from scratch.
- Impact: Faster identification of potential new treatments for various diseases, including those with limited research or treatment options.
5. Automated Synthesis and Lab Automation
- Trend: Integration of AI with robotics and automation technologies to streamline chemical synthesis and laboratory experiments.
- Impact: Increased efficiency and accuracy in compound synthesis and testing, reducing the time and cost associated with drug discovery.
6. Real-world data and AI
- Trend: Utilizing real-world data (RWD) from electronic health records, patient registries, and wearable devices in conjunction with AI to inform drug discovery and development.
- Impact: More relevant and applicable insights into drug efficacy and safety in diverse patient populations, enhancing the relevance and precision of clinical trials.
7. AI-Enhanced Drug Design and Virtual Screening
- Trend: Advances in AI algorithms to improve virtual screening techniques and drug design processes, including more accurate prediction of drug-target interactions and side effects.
- Impact: More efficient and accurate identification of promising drug candidates and a better understanding of potential risks.
8. Integration with Quantum Computing
- Trend: Incorporation of quantum computing to handle complex molecular simulations and large-scale data analyses that are challenging for classical computers.
- Impact: Accelerated drug discovery processes, with the potential for breakthroughs in understanding molecular interactions and optimizing drug properties.
9. Collaborative AI Platforms
- Trend: The emergence of collaborative AI platforms that bring together researchers, pharmaceutical companies, and AI developers to share data, tools, and insights.
- Impact: Enhanced collaboration and data sharing, leading to more comprehensive and faster drug discovery efforts.
10. Ethical and Regulatory Considerations
- Trend: Growing focus on the ethical and regulatory implications of AI in drug discovery, including data privacy, algorithmic bias, and transparency.
- Impact: Development of guidelines and best practices to ensure responsible use of AI technologies in drug discovery and address potential concerns related to fairness and security.
11. AI-Driven Drug Safety and Pharmacovigilance
- Trend: Application of AI to monitor and analyze drug safety data from clinical trials and post-marketing surveillance.
- Impact: Early detection of adverse effects and improved understanding of long-term drug safety, leading to better risk management and patient safety.
The future of AI-driven drug discovery is characterized by greater integration of diverse data sources, advanced generative models, and the application of cutting-edge technologies like quantum computing. These trends promise to accelerate drug development, enhance personalization, and improve overall drug discovery processes. As AI continues to evolve, its role in transforming the pharmaceutical industry will likely expand, offering new opportunities for innovation and efficiency in developing effective treatments.
Conclusion
In conclusion, generative AI is transforming the future of drug discovery by streamlining processes, improving accuracy, and reducing the cost and time associated with developing new treatments. Its ability to analyze massive datasets, simulate drug interactions, and suggest novel molecular structures is accelerating research breakthroughs that were once thought impossible. By enabling faster preclinical research and enhancing drug design precision, generative AI is boosting the pharmaceutical industry’s ability to respond to health challenges, including rare diseases and antibiotic-resistant strains.
Moreover, its capacity to evolve and adapt makes it a powerful tool that can keep pace with the ever-changing landscape of medical needs. As AI technology continues to advance, its role in drug discovery will likely expand, paving the way for innovative treatments and more personalized therapies. The integration of AI into drug discovery is not just a technological advancement; it represents a paradigm shift that could revolutionize how we understand, treat, and prevent diseases, making healthcare more efficient and effective for future generations.

How Generative AI is Transforming Drug Discovery: A Deep Dive was originally published in Coinmonks on Medium, where people are continuing the conversation by highlighting and responding to this story.